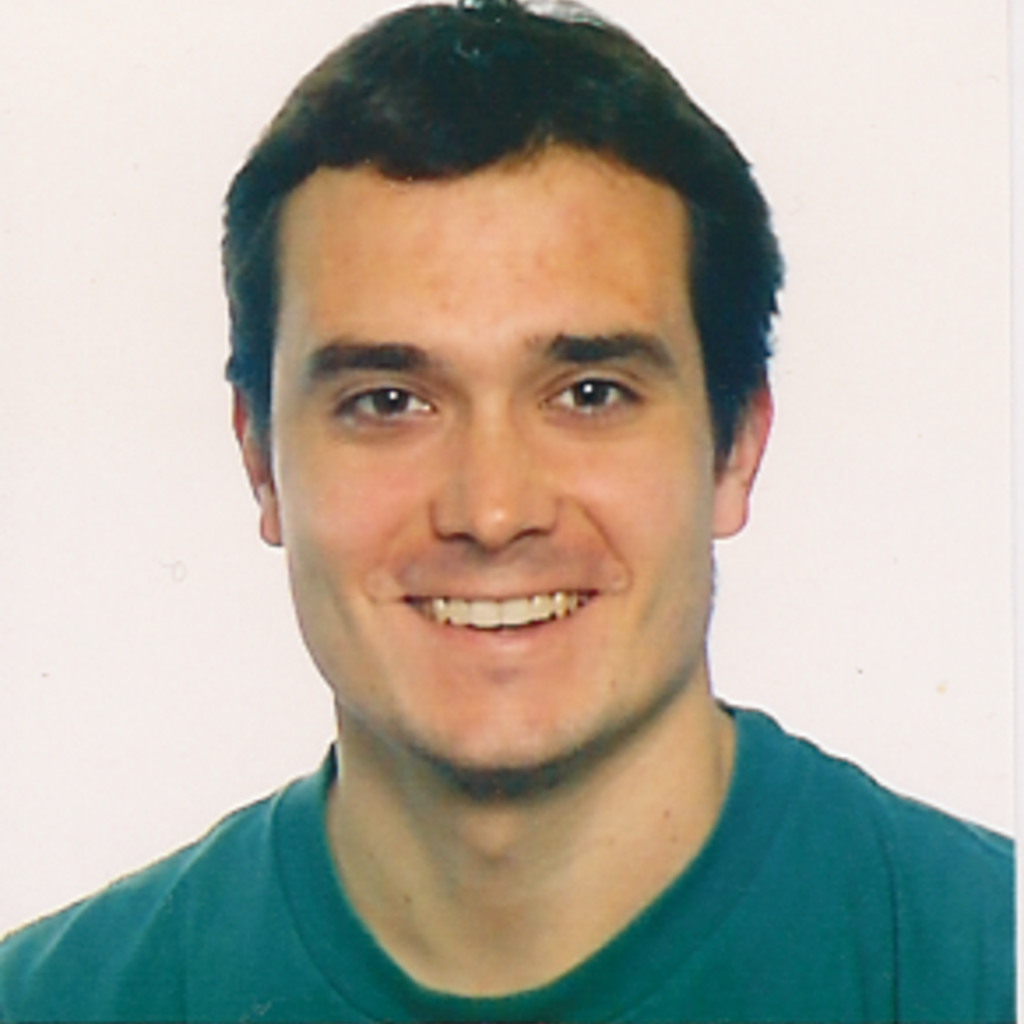
Speaker
Iñigo Urteaga
Abstract
Mobile health (mHealth) apps, such as menstrual trackers, provide a rich source of self-reported observations: they provide day-to-day health indicators and behaviors, which can help shed light onto an individual's wellness and health over time. However, self-tracked data collected via mHealth apps have questionable reliability, as they hinge on user adherence. Because mHealth app users may skip tracking relevant health information, disentangling physiological patterns from tracking behaviors is necessary.
In this talk, I will describe how probabilistic machine learning can provide robust predictive models of self-tracking data in the context of women's health in general, and the menstrual cycle in particular. Namely, I will present statistical generative models that accommodate mHealth self-tracked variables that are subject to per-user missingness patterns, to provide personalized and accurate predictions of next cycle start date.
On the way, I will showcase the importance of disentangling physiological patterns from self-tracking adherence to attain informative predictions with mHealth data, as well as how flexible statistical models enable well-calibrated predictions that accommodate the idiosyncrasies and uncertainties of mHealth self-tracked data.
Bio
I am an Associate Research Scientist in the Applied Physics and Mathematics department at Columbia University and affiliated with its Data Science Institute. I have specialized in statistical machine learning, computational Bayesian statistics, approximate inference methods, and sequential decision processes.
My research focuses not only on the development of Bayesian probabilistic models and algorithms, but also on their application to a wide range of disciplines. I am currently working on descriptive, predictive, and prescriptive modeling with applications in science, engineering and healthcare.
I was a data-science postdoc at Columbia University from 2016 to 2018 working with Prof. Chris Wiggins and Prof. Noémie Elhadad on statistical machine learning for healthcare data, in the context of electronic health records and self-tracked data.
I completed my Ph.D. in Electrical Engineering at Stony Brook University in 2016 under the supervision of Prof. Petar M. Djurić. My dissertation is entitled "Sequential Monte Carlo methods for inference and prediction of time-series". My research attracted interest in econometrics (for prediction in stochastic volatility models) and biomedicine (for the study of fetal heart-rate signals).
I previously obtained my degree in telecommunications engineering from the UPV/EHU Faculty of Engineering in Bilbao, Spain, and worked in Research and Development projects at Tecnalia.